Amirali Amirsoleimani, assistant professor in the Electrical Engineering & Computer Science Department at York University’s Lassonde School of Engineering is leading efforts to advance neuromorphic computing, which aims to develop specialized software and hardware modelled after the human brain, mimicking its biological structure and function to establish computer systems with human intelligence at their core.
Like the human brain, which uses a complex system of neurons and other structures to perform various functions, neuromorphic computing systems use an expansive network of artificial neurons to receive and transmit signals, allowing for humanistic skills such as the ability to learn from and adapt to changing stimuli. These systems also replicate the brain’s ability to perform advanced tasks with minimal energy and remain functional when partially damaged, supporting energy-efficient computing systems that are resilient against component failure.
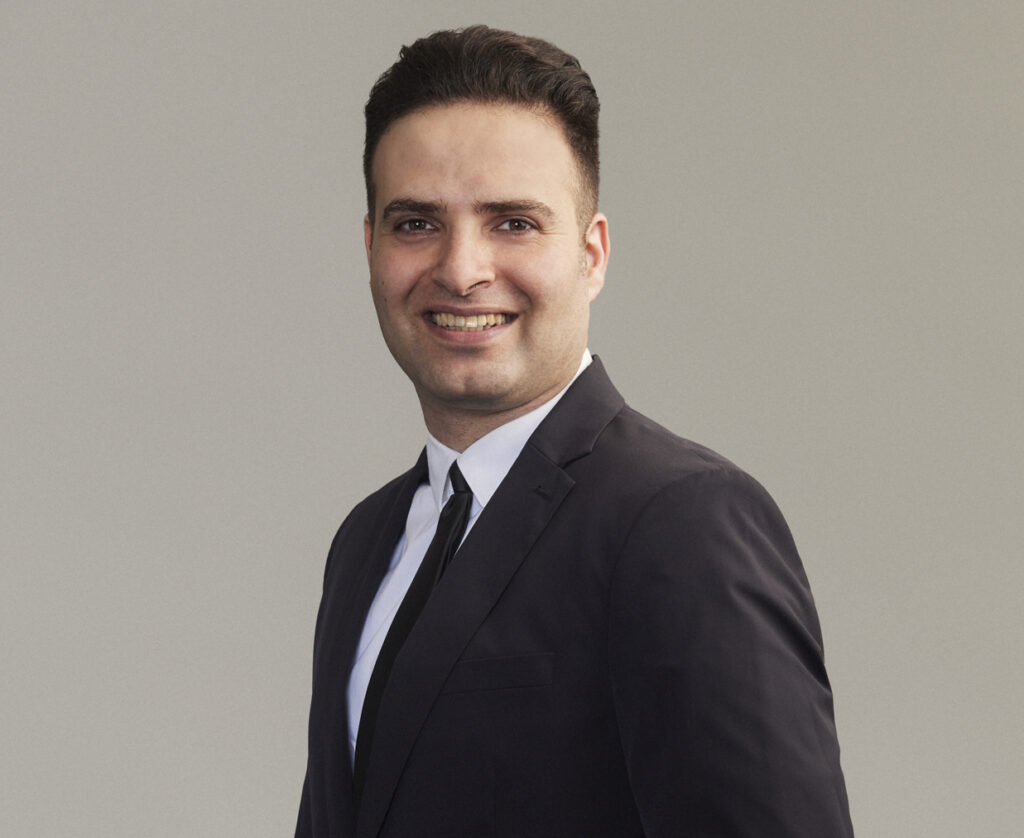
“By building computing systems that mimic the human brain, we can improve computing efficiency and also extend applications to health care. We need to change the way we’re computing,” says Amirsoleimani.
His work has explored the use and optimization of numerous hardware technologies to support a future, global transition from traditional to neuromorphic computing systems. This includes using emerging technologies to build spiking neural accelerators, which can reduce energy consumption and footprint, as well as increase throughput.
Amirsoleimani’s intent is to advance this emerging research field focused on building hardware for neuromorphic computing systems, which can be overlooked during the current wave of artificial intelligence (AI) advancements. “The AI side of research is very saturated, but not many people work on the hardware side,” he says. “We want to build hardware to support the design of novel computing systems.”
In a recent project, Amirsoleimani and his research team proposed a novel system for epileptic seizure detection and prediction. As the underlying mechanism of epilepsy is not entirely understood, experimental methods of treatment require accurate detection and prediction systems. Researchers have explored the use of electroencephalograms (EEG) and human intracranial electroencephalograms (iEEG) to monitor electrical activity in the brain, coupled with machine learning (ML) algorithms to classify seizures; however, these methods have proven to be tedious and inconvenient.
Amirsoleimani’s project explored the use of a convolutional neural network (CNN) based on in-memory computing (IMC) resistive random-access memory (RRAM) architecture, with analog crossbars. This dual-function architecture allowed for data storage and computation, and resulted in patient data being collected from EEGs and iEEGs in a way that the system effectively detected and predicted epileptic seizures with 97.5 to 99.8 per cent accuracy.
With promising benefits and applications of these intelligent computing systems, Amirsoleimani continues to work towards his purposeful research goals, while tackling the many obstacles that overwhelm the field of neuromorphic computing. “You have to be very flexible with this research; there is a lot of trial and error involved,” he says. “The world doesn’t even know exactly how the human brain works yet, so it can be difficult to develop computing systems modelled after something that isn’t completely understood.”
Amirsoleimani’s Lab for Computing Research and Innovation (LCRAIN) is open to interested undergraduate and graduate students looking to change the future of computing. Learn more about LCRAIN and research opportunities available in the lab.